Annual Schedule
The annual schedule starts in April 2022 from Fundamental I, followed by Application II for follow-up and level-up. In addition, joint research and on-the-job training (internship) will be offered to individuals after consulting with host institutions.
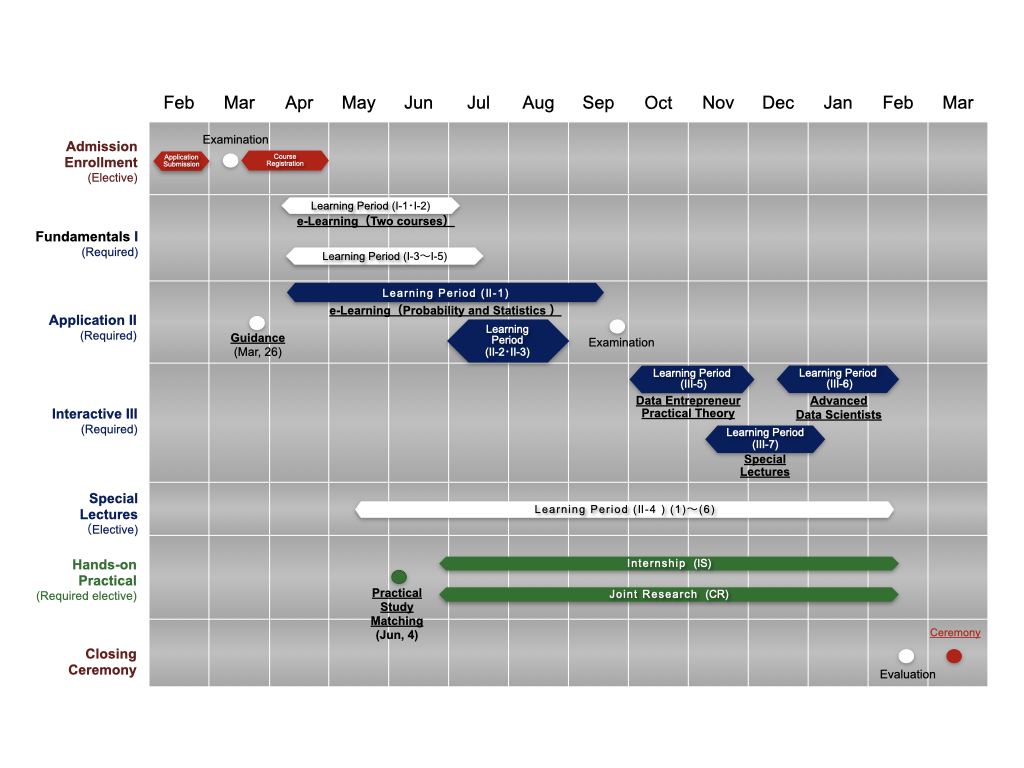
Completion Requirements
For completion requirements, please check the courses and items required for each position.
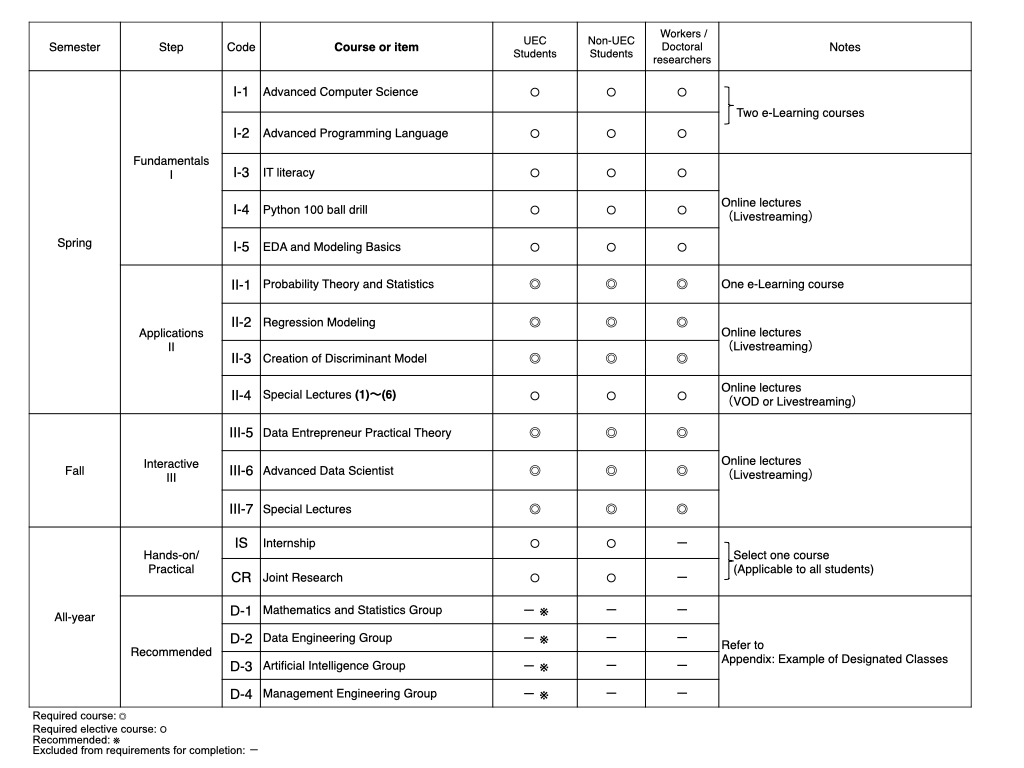
Appendix: Example of Designated Classes (The University of Electro-Communications)
Non-degree students can attend with additional fees
Mathematical and Statistical Group | Data Engineering Group | Artificial Intelligence Group | Management Engineering Group |
Fundamentals of Analysis | Advanced Topics on Discrete Information Structure | Advanced Study for Theoretical Computer Science | Management Planning |
Advanced Topics in Analysis | Foundation of Discrete Optimization | Fundamentals of Algorithm Theory | Management Principles and Practices |
Fundamentals of Applied Analysis | Foundation of Continuous Optimization | Applied Algorithms | Advanced Management Systems Engineering |
Mathematical Analysis | Topics on Theory of Computation | Advanced Topics on Algorithmic Engineering | Management Information Systems |
Fundamentals of Information Theory | Fundamentals of Computer Architecture | Advanced Topics in Machine Learning | Project-based Learning of Practical Information System |
Advanced Information Theory | Advanced Computer Science | Advanced Intelligent Information Systems | Advanced Intellectual Property |
Advanced Theory on Information Data Analysis | Parallel Processing I | Game theory | Advanced Engineering Development |
Fundamentals of Mathematical Statistics | Parallel Processing II | Advanced Theory of Software Sciences | Advanced Lectures on Venture Business |
Data Mining | Advanced Theory of Software Sciences | Advanced Topics on Intelligent Soft Computing Systems | Advanced Service Science |
Advanced Theory on Bayesian Artificial Intelligence | Advanced Progamming Languages: Concepts and Implementation | Artificial Intelligence and Complex Network | Software Quality |
Advanced Statistical Machine Learning | Advanced Topics on System Software | Advanced Image Recognition Systems | Introduction to Information Technologies |
Advanced Topics in Data Analysis Optimization | Fundamentals of Practical Software Development | Advanced Cognitive Sciences | Advanced Financial Engineering |
Fundamental of Geometry | Practical Software Development II | Learning Informatics | Fundamentals of Accounting Information Systems |
Advanced Topics of Geometry | Practical Software Development III | Topics on Cognitive processing | Advanced Theory of Systems Reliability |
Advanced Topics on Information Geometry | Principles of Data Engineering 1 | Advanced Topics in Language and Cognitive Systems | Risk management |
Fundamentals of Algebra | Principles of Data Engineering 2 | Advanced Topics on Intelligent Robotics | Advanced Manufacturing Systems Engineering |
Advanced Topics in Algebra | Advanced System Design 2 | Advanced topics in perceptual system | Advanced Intelligent Production System |
Modern Algebra | Advanced System Design 1 | Advanced Data and Knowledge Engineering | Data Entrepreneur Practical Theory |
| | | Advanced Data Scientist |
Fundamentals I
e-Learning (elective)
Anyone enrolled in the program can participate. In this elective course, participants watch video materials and answer prescribed assignments.
(I-1) Advanced Computer Science
The course covers computer science basics, which is essential for understanding the various data science methods. After an initial introduction to computer science, students will learn about algorithms’ concepts to give an overview of the calculation algorithms for the greatest common factor, factorization, and power. After studying congruence formulas, finite fields, and Fermat’s little theorem, we explain their applications to RSA public-key cryptography and digital signatures. We discuss small-world phenomena in graph theory after lecturing on the breadth-first search, paths and connected components, and rooted trees. Finally, we lecture on the basics of computational complexity theory. Specifically, the concepts of computational time measurement, polynomial time algorithms, and explosive increases in computational time are studied, We introduce the P versus NP problem, bitcoin, and quantum computer topics after lecturing on the concepts of how to measure computation time, polynomial-time algorithms, and an explosive increase in computation time.
10 sessions in total
- 1. Algorithm #1: What is computer science? What is an algorithm? Calculation of the greatest common factor and Euclid’s algorithm
- 2. Algorithm #2: Factoring algorithm, exponentiation calculation, power calculation time, and factoring difficulty
- 3. Theory of integers #1: Congruence formulas, algebras of congruence formulas, and fundamental problems of algebra
- 4. Theory of integers #2: Euclid’s algorithm, theorems about greatest common factors, finite fields, and Fermat’s little theorem
- 5. Public-key cryptography: Public-key cryptography, RSA public-key cryptography, and electronic signatures
- 6. Graph theory #1: Graph definition, paths and connectivity, and rooted trees
- 7. Graph theory #2: Graph breadth-first search, connected components, and complex network analysis
- 8. Computational complexity theory #1: How to measure computation time, polynomial-time algorithms, and an explosive increase in computation time
- 9. Computational complexity theory #2: Clique problem, clique problem complexity, and P versus NP problem
- 10. Computational complexity theory #3: NP-complete problems, bitcoin, and quantum computers
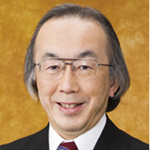
Lecturer
NISHINO Tetsuro
(I-2) Advanced Programming Languages
This course lectures on the basics of the Python programming language required for data science. Students acquire the language through practice. After learning the Python programming language’s grammar, we move on to the control scripts and object-oriented concepts and notation. After the modules for data analysis, we study visualization and conduct a general exercise. Learning the Python programming language framework leads to practices in the Advanced Data Scientists in the second semester.
10 sessions in total
- 1. Building a Python experimental environment
- 2. Try Python: Data structures of interpreter shells and arithmetic processing; variables, strings, and lists;
- 3. Python as a script #1: From the interpreter to the script, how to write and indent control
- 4. Python as a script #2: How to write and use functions and the scope of variables
- 5. Python as an object-oriented language: How to write and use classes and generate instances
- 6. Module overview and import #1: Explanation of standard modules, interfaces of the OS, string pattern matching, math, Internet access, date and time, data compression, performance measurement, and quality control
- 7. Module overview and import #2: Introduction to the outline of NumPy, SciPy, Matplotlib, and pandas modules used for scientific and engineering calculations
- 8. NumPy overview and visualization with Matplotlib
- 9. SciPy overview and data analysis
- 10. Examination
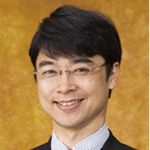
Lecturer
SHOUNO Hayaru
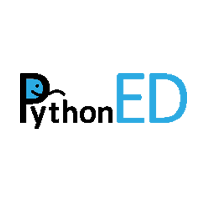
Courses offered by the Python Engineer Development Association
- Manabu Terada, Advisory Director of the Association, examination question supervision and community support
Online Courses (elective)
Anyone enrolled in the program can participate. In this elective course, lectures are given by Zoom, and questions are answered via Slack.
(I-3) IT Literacy (live streaming)
Participants acquire basic literacy to work with data.
- ・Access to remote servers via SSH communication
- ・Character Codes and Normal Expressions
- ・Introduction to TCP/IP and HTML
- ・Using Git and Git Hub
- ・Introduction to SQL
(I-4) Python “100 ball drill” (live streaming)
Self-teaching with the book “Data Science 100 ball drill (Structured Data Processing Edition)” published by the Data Scientists Association of Japan. Questions are accepted via Slack.
(I-5) EDA and Modeling Basics (live streaming)
The class covers the basics of data aggregation, visualization, and machine learning using Python.
- ・Data aggregation using pandas
- ・Data visualization and data structure
- ・Model Accuracy and Evaluation
- ・Introduction to Discriminant Models (Titanic Data)
- ・Introduction to regression modeling (J-League attendance forecasting)
Applications Ⅱ
e-Learning (required)
Participants watch video materials and answer prescribed questions.
(II-1) Probability and Statistics
Probability and statistics are the foundation of scientific knowledge in the three skill areas of data science and are essential for studying machine learning and AI. Alternatively, it is also a means of learning how to think mathematically about various phenomena.
In the first part, you will learn about random variables. The second part will cover basic statistical techniques. And lastly, in the third part, we will use the knowledge gained to explain the methods employed in actual data analysis from a statistical standpoint.
While keeping the necessary fundamentals in check, the lectures at the end of each section will provide an overview of the more advanced contents for subsequent study.
Part I: Random Variables
1. Basics of Probability
2. Continuous-valued Random Variables
3. Multidimensional Random Variables
4. Law of Large Numbers and Central Limit Theorem
Part II: Statistical Estimation and Testing
5. Probability Distribution of Sample
6. Point Estimation and Interval Estimation
7. Statistical Hypothesis Test
8. Information Theory and Statistics
Part III: Statistical Modeling
9. Linear Regression Analysis
10. Logistic Regression Analysis
11. Time Series Analysis
12. Bayesian Statistics and Modeling
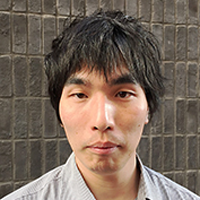
Lecturer
HARADA Kei
Online Courses (required)
The lectures are via Zoom, and questions are answered via Slack.
(II-2) Regression Modeling (live streaming)
Through studying Washington DC’s real estate property price forecasting, students learn the basics of regression analysis. Participants in the lecture form groups, and each group competes on the accuracy of their models. Lectures and hands-on practice are the main focus.
(II-3) Creation of Discriminant Modeling (live streaming)
Participants create a binary classification model from purchasing behavior in retail stores. The model will discriminate between consumers who show interest in certain products and who do not. The datasets used in this lecture were obtained initially by Konica Minolta, a participating organization in the consortium, and are efficient data directly related to marketing.
(II-4) Special Lectures (elective)
This is the elective course and anyone enrolled in the program can participate. The lectures are delivered on-demand or online via Zoom. Questions are answered via Slack.
(1) Causal Inferences (on-demand streaming)
Participants will learn causal inference basics from lecture materials.
(2) Recommendation Model (on-demand streaming)
Participants will make a recommendation model using the lecture and exercise materials. Most data are public, but some data are provided by supporting corporations.
(3) Natural Language Processing – Basic (on-demand streaming)
After explaining pre-processing, which is the base for working with natural language processing, participants will be guided through the applications. The lecture will use slide materials and a notebook for exercise.
(4) Natural Language Processing – Advanced (on-demand streaming)
This course covers topic models with LDA, Word2Vec, and BERT as advanced subjects of natural language processing basic. The lecture will use slide materials and a notebook for exercise.
(5) Fundamentals of Deep Learning (on-demand streaming)
The course objective is to acquire universal ideas that can be used for a long time in this field, which is evolving at a rapid pace. Through lecture materials and exercises, participants will deepen their understanding of the theoretical background by transcribing it from mathematical formulas to codes.
(6) 2022 Special lectures (live streaming)
Coming soon.
Interactive III
Online Courses (required)
This is the live-streaming online course by zoom.
(III-5) Data Entrepreneur Practical Theory
The course focuses on business theories for handling data science in business, from leading researchers, engineers, and executives in various industries. After learning best practices from business leaders, each participant will present a business plan using data science.
Academic Year 2021:15 sessions in total
1. The Workstyle of Data Scientist as a Freelance or entrepreneur – AKAMA Satoru, Freelance
2. Data Science of Kaggle and On-site – SAKATA Takashi, Panasonic Corporation
3. A start-up case study of a location data analysis business – ISHIKAWA Yutaka, Knightley Inc.
4. From Data to Transformation – IWAKURA Tomoya, Fujitsu Laboratories, Fujitsu Limited
5. Analytics to Increase Customer Value – NISHIMAKI Yoichiro, IBM Japan, Ltd.
6. Examples of Big-data Application – MORIYA Toshio, Hitachi, Ltd.
7-9.Creating a new business by design thinking To maake a Chatbot Service – NISHINO Tetsuro, The University of Electro-Communications
10. Business Decision Making by Data Analysis – WADA Yoichiro, Data4Cʼs K.K.
11. Business Model Creation in the IoT Era – SHIMADA Keiichiro, R&D Center, Sony Group Corporation
12. About Recruiting Data Scientist – HARADA Kei, DeNA Co., Ltd.
Past Curriculum(Japanese only)
・Academic Year 2021: 15 sessions
・Academic Year 2020: 15 sessions
・Academic Year 2019: 15 sessions
・Academic Year 2018: 15 sessions
・Academic Year 2017: 15 sessions
・Academic Year 2016: 10 sessions
・Academic Year 2015: 3 sessions
(III-7) Special Lecture: Kaggle
Compared to “data science” terminology, “machine learning modeling” is relatively limited coverage. However, many things cannot be understood even for model building without actually looking at and processing the data. Therefore, there is unique know-how outside of textbooks. It is essential to accumulate practical experience, look at the data yourself, and write your own code. By participating in competitions such as Kaggle, students can gain pseudo-practical experience before taking up such a position. Each session consists of one lecture and two exercises, and participants will acquire model-building skills through competition-style exercises.
Past Curriculum
・Academic Year 2021: Special Kaggle lectures, total 15 sessions
1-3. Step-by-step Model Building
4-6. Joining and Aggregating Tables
7-9. Multi-class Classification and Ensembles
10-12. Handling Time Series Data
13-15. Table data including Natural Language
Practical Learning
Internship (On-the-job Training)
This program is designed for graduate students to experience practical problem-solving work by participating in an internship at a company. The University of Electro-Communications’ Center for Industry-Academia-Government Collaboration, the Internship Promotion Office of the Career Education Subcommittee, Division of General Education, and URA staff will assist the students in deciding which companies to accept and what internship program to pursue. The target year, internship period, and research/technical development topics will be determined flexibly in response to individual consultation. Students who wish to participate in the program will be required to submit a request form. Insurance coverage, company confidentiality, and travel expenses will be covered in the same manner as the conventional internship program.
Reference: http://www.uec.ac.jp/career/career/procedure.html
The internship granted for two credits by the Career Education Subcommittee’s Internship Promotion Office is, in principle, a summer internship of at least 90 hours for first-year master’s students. The report and performance evaluation may be used to apply for completion requirements for this program.
Reference: http://www.uec.ac.jp/career/career/internship.html
Joint Research
This program is intended for graduate students to experience practical problem-solving methods and effects by getting involved in joint research between their laboratories and companies.
Depending on the content and scale of the assignment, the implementation format will vary and will be discussed on an individual basis. In practice, specific assignments are set through guidance and consultation meetings scheduled at the beginning and in the middle of the academic year.
The University of Electro-Communications is home to a large number of faculty and researchers with outstanding achievements in the fields of advanced science and technology, focusing on electronics, information and communications, computers, mechatronics, intelligent robotics, biotechnology, laser and optical technologies, new materials, etc. More than 300 professors from science and engineering to humanities participate in joint research in their respective fields of expertise.
The program will focus on setting up assignments in information and communication, particularly in AI, IoT, big data, and cybersecurity.
Reference: http://www.crc.uec.ac.jp/institution/